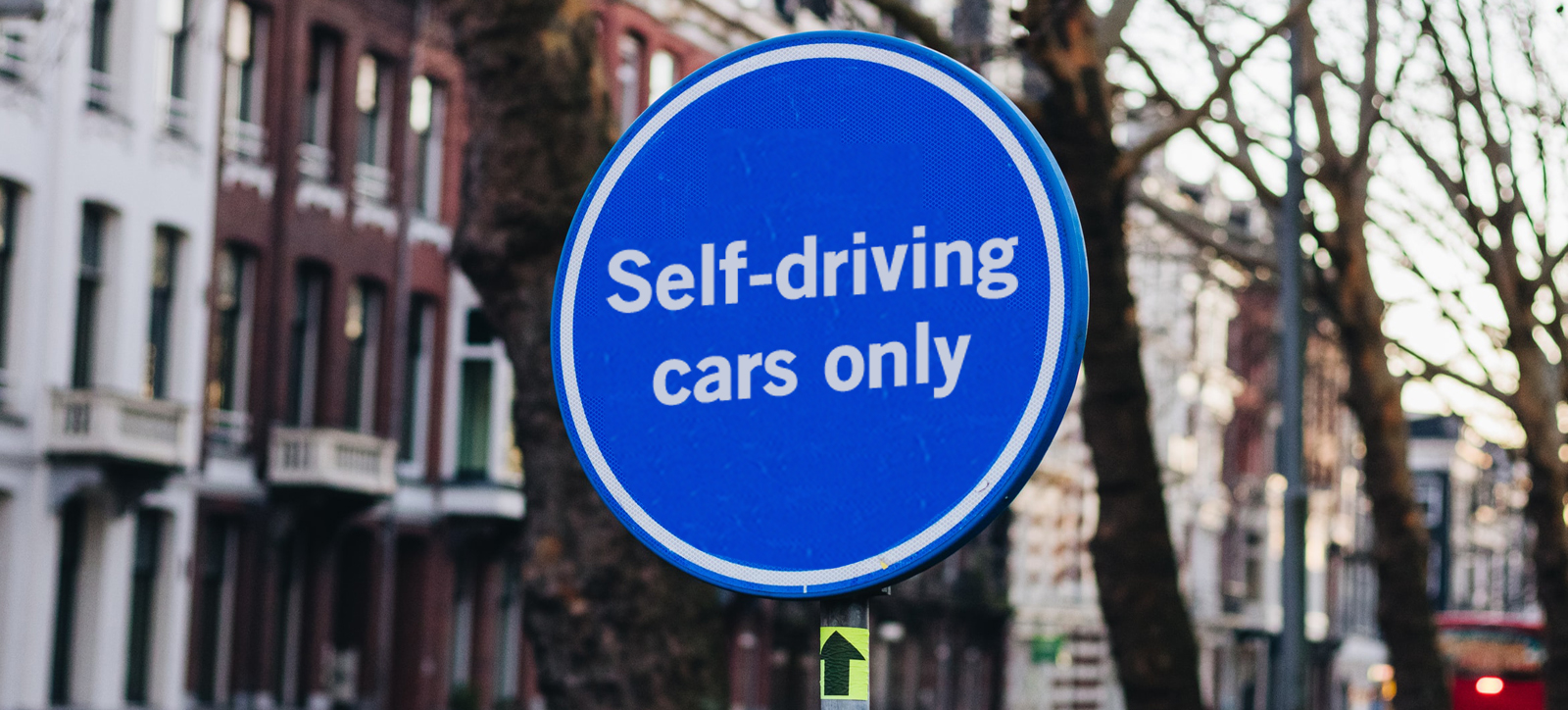
Heading to buy my groceries in narrow city streets, I have to wend my way past dozens of parked cars, hoping my wing mirrors will come out intact.
It is a complicated process, stopping behind rows of parked cars, waiting for other drivers coming the other way, wondering if there may be any more, taking the chance to come out, then deciding whether the oncoming traffic is going to let me through or whether I should give way.
Sometimes, if I am in a hurry, I will be slightly bolder and just keep driving, hoping the oncoming traffic will wait and not decide to ‘go for it’ too. If they do then I have to dart behind another parked car, or we both stop and judge together what we should do next - perhaps we can squeeze past each other after all.
This process usually works. Somehow, we all get to where we want to go and escape with no scrapes or scratches. Occasionally there is a bump or traffic comes to a standstill as the negotiations turn into deadlock and somebody needs to reverse out of the way; but on the whole this chaotic, improvised negotiation works remarkably well.
But imagine trying to model this traffic negotiation, writing down the steps of thinking and acting that we go through. It gets hideously convoluted - and each day presents new challenges: different layouts of road, cars, pedestrians, bicycles, prams and, of course, differing weather.
Then try the challenge of turning this model into a computer algorithm, that an autonomous car might follow. What are the rules and signals being used? There are so many and the trouble is they evolve.
If it is not clear which car should go first, then drivers will often solve the problem by communicating. But this creates further challenges - how do we capture the principles by which our varied headlight-flashing, waving, pointing, nodding, honking or just smiling can be interpreted?
A self-driving car’s cameras won’t be good enough to see the smiling, pointing or nodding. It might be programmed that flashing lights means to go (though this will work better in some countries than others); but flashing lights can also mean ‘hello!’ (when recognising a friend), or ‘watch out’, or ‘there is something wrong with your car’ or that 'I am angry about your terrible driving!' The context usually gives the answer, so that implies we might need a rule for each context; and there is an apparently limitless number of different contexts.
My colleagues and I have been trying to model this traffic negotiation over the last few years, with the long term aim of helping so that autonomous vehicles, human motorists, cyclists and pedestrians can negotiate our roads safely. It involves addressing fundamental questions at the frontiers of cognitive science, questions of human behaviour that have not been fully answered.
These challenges involve familiar issues in perception and control (interpreting the road environment, steering the car smoothly and safely), but less obviously, and arguably far more difficult, questions concerning the cognitive foundations of social interaction.
We have used a new approach to social interaction that we have been developing to understand how people communicate and interact, called ‘virtual bargaining’, a variation of mathematical game theory that establishes how the ‘unwritten rules’ of many social interactions are established.
How will autonomous cars negotiate the traffic?
The core idea is that, when two people are doing a task together (whether lifting and moving a heavy table, playing doubles tennis, or figuring out who goes first in congested traffic), then both people tend to favour the option that they would have chosen had they explicitly been able to bargain. Of course, often there is no time or opportunity for actual bargaining, but if it is obvious to both of us what the bargaining would conclude, then actual bargaining is unnecessary. A virtual ‘imagined’ bargain is sufficient.
So when confronting another vehicle on a narrow country lane, it may be ‘obvious’ that, because one of us has only to reverse a short distance, then an explicit discussion would conclude that this person should retreat. If that vehicle is a ministerial car or a tank, it may seem obvious that this vehicle would ‘win’ any negotiation, whatever the layout. Virtual bargaining will ensure that both vehicles do what would be agreed, without having to labour through any actual negotiation.
How well autonomous cars can solve these traffic negotiations with human drivers, cyclists and pedestrians is vital for their introduction onto our roads. Unless we can model this bargaining on the roads so autonomous cars are socially sophisticated, autonomous vehicles could be dangerous, as self-driving vehicles mis-read the cues and cause an accident; or simply annoying, as they have to be excessively cautious until the situation is resolved, perhaps causing frustration for the passenger, and even a tailback in the rush hour traffic.
Even explicit negotiation is generally extremely difficult to model, despite decades of intensive analysis in game theory, management, political science, and psychology; so to model an unspoken interaction with various highly ambiguous cues is extremely complicated. It is the motoring equivalent of passing the Alan Turing test, which challenges a machine’s ability to exhibit behaviour indistinguishable to a human.
We have all seen impressive videos of Tesla drivers switching on autopilot while on motorways or the multi-laned freeways in the US, where they happily sit reading as the car speeds along by itself. And the problem of sensing the surroundings and other road users appears to be yielding impressive progress, but the challenge of moving these cars into the tighter confines of towns and cities where traffic ‘negotiation’ is commonplace has scarcely been addressed.
In fact, the rate of progress on this challenge within the cognitive sciences may prove a decisive limiting factor in the development of autonomous vehicles.
Can AI make autonomous cars safe for our roads?
These problems are, I believe, too hard to solve with current algorithms. To solve these problems as a ‘virtual bargainer’, means thinking from the other person’s point of view and coming to the same conclusion - that is very difficult as the differences are very subtle.
Every time an extra complication is added, such as a pedestrian, then all the calculations have to be re-programmed. Every time the environment changes or even the weather - with people holding umbrellas, and then what does waving an umbrella mean - requires a new set of algorithms. There is not one set of algorithms that will solve it because there are limitless possibilities and combinations.
Could the fast-developing field of machine learning be the solution? I suspect it will be difficult as machine learning requires huge amounts of data to learn from and developing the data from the vast array of scenarios on our roads is another complex problem. Differentiating between pictures of dogs and cats requires thousands of photos for AI to learn from, and how much more complex is the space of possible traffic negotiation scenarios?
Car manufacturers and tech giants are all grappling with these problems to allow autonomous vehicles to move among us. But I am beginning to think that the the best way forward may be to avoid, rather than to confront, the problem: to give autonomous cars their own roads and lanes.
To mix them with human drivers on our complicated and cluttered roads seems too overwhelming and dangerous. Already a pedestrian has been killed by Uber’s self-driving car as its perception system became confused by the woman pushing her bike across the road - traffic negotiation presents another hazardous and perplexing situation.
After some time these spaces can be gradually increased until all our vehicles are autonomous - what we’ll be creating is something more like a flexible train or tram system (though with no rails). Self-driving cars offer an exciting future with many possibilities, but autonomous vehicles can become a reality most easily if the rest of us road users keep out of their way.
Further reading:
Chater, N., Misyak, J. B., Watson, D. G., Griffiths, N. and Mouzakitis, A. (2018) "Negotiating the traffic: can cognitive science help make autonomous vehicles a reality?", Trends in Cognitive Sciences, 22, 2, 93-95.
Misyak, J. B. and Chater, N. (2014) "Virtual bargaining: a theory of social decision-making", Philosophical Transactions of the Royal Society: B. Biological Sciences, Volume 369, Number 1655.
Misyak, J. B., Melkonyan, T. A., Zeitoun, H. and Chater, N. (2014) "Unwritten rules: virtual bargaining underpins social interaction, culture, and society", Trends in Cognitive Sciences, Volume 18, Number 10, 512-519
Nick Chater is Professor of Behavioural Science and author of The Mind is Flat: The Illusion of Mental Depth and The Improvised Mind. He teaches Behaviroual Science in Practice for senior managers and executives.
For more articles like this download Core magazine here.