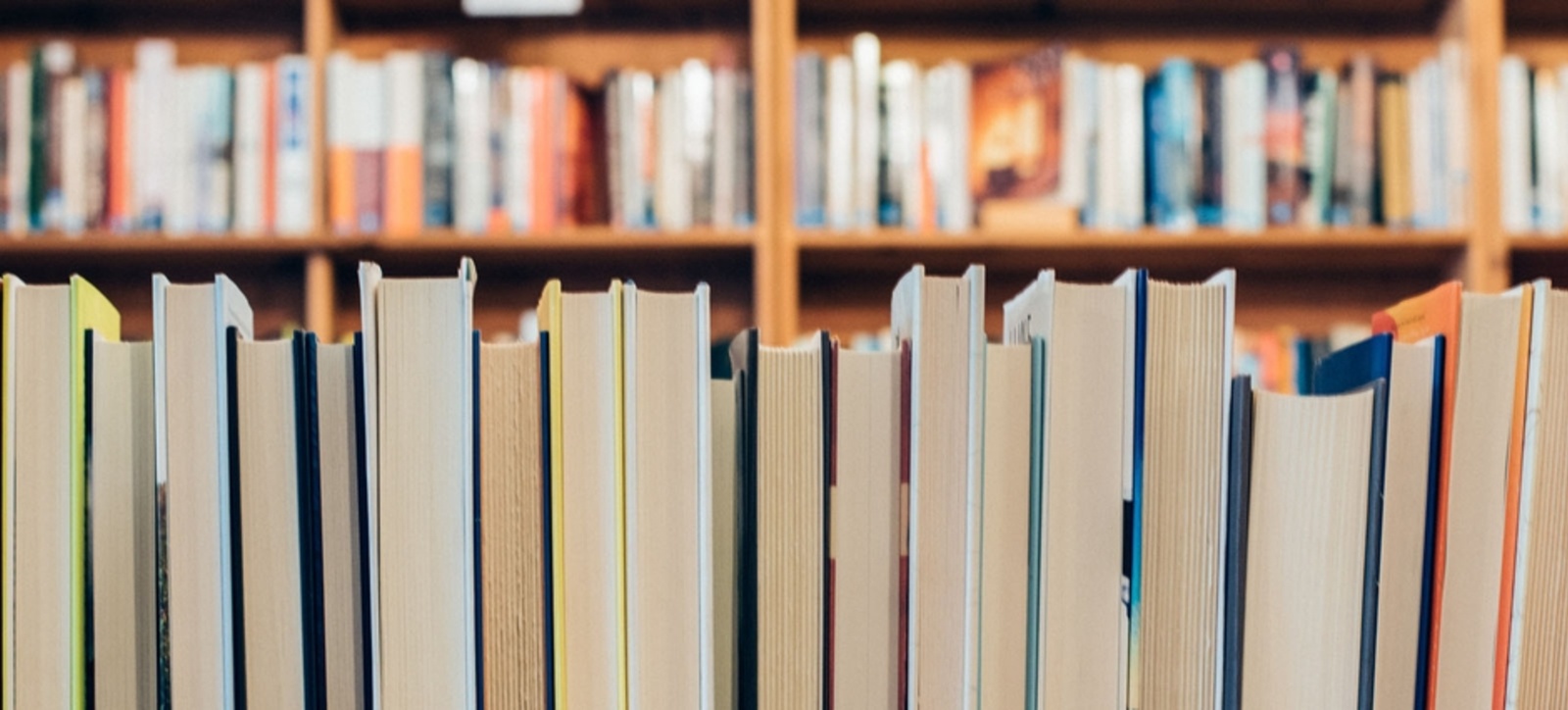
Has somebody made you aware that the algorithms and the data that drive machine learning - and Artificial Intelligence (AI) more broadly - have the potential to repeat past biases?
Have you been told how Amazon stopped using their AI recruiting tool because it showed a bias against women because training data looked at past successes?
Despite the biases of AI, Amazon and other tech firms continue to benefit from developing AI-based solutions. And so should all businesses.
The following five suggested reads aim to raise awareness on the topic of trustworthy AI, looking at the principles and then some applied examples, with a reminder that the ethical merits of an AI-based tool should be compared to the non-AI-based status quo.
1 The global landscape of AI ethics guidelines
By Anna Jobin, Marcello Ienca, and Effy Vayena. Nature Machine Intelligence 2019.
There are no single ethical requirements, technical standards or best practices that achieve ethical and trustworthy AI.
Jobin and her colleagues discuss in their article a global convergence around five ethical principles: transparency, justice and fairness, non-maleficence, responsibility, and privacy.
By doing so the authors manage to familiarise readers with broad ethical issues around AI and the different interpretations of the ethical requirements.
2 Ethics guidelines for trustworthy AI
By the European Commission 2019.
Organisations might want to apply specific guidelines as well as demonstrate how they apply ethical principles.
To develop trustworthy AI and to demonstrate appropriate implementation strategies throughout a project's life cycle I recommend following a specific set of guidelines, such as the EU Ethics guidelines for trustworthy AI.
According to the guidelines trustworthy AI should be: lawful, ethical and robust. Specifically, the EU guidelines recommend seven key requirements that AI systems should meet in order to be deemed trustworthy.
To help organisations verify the application of each of the key requirements the EU provides a detailed assessment list can be found here, while consultants McKinsey have written an analysis of the impact for businesses.
Jobin, Ience and Vayena's paper compares their five broad areas outlined with the EU guidelines. Bu please note that because AI is a relatively new field, the guidelines are not yet compulsory EU regulation, unlike the General Data Protection Regulation (GDPR), for example.
3 Machine behaviour
By Iyad Rahwan et al. Nature 2019.
Clear guidelines tend to give the impression of an easy to follow ‘tick list’, however, this is far from true for AI Ethics.
This paper illustrates some of the multi-faceted issues relating to AI ethics. The authors explain, for example, how imperfections in data can significantly impact results.
They also discuss how on the one hand source code and training data are frequently proprietary to the developers and thus hidden to users, while on the other hand open source programming algorithms can be too complex and too difficult to interpret.
Alternatively, the algorithms underpinning particular AI-based tools can be quite simple, however, the results might be very complex and again too difficult if users need them to make adequate judgements.
4 Rho AI: Leveraging artificial intelligence to address climate change: Financing, Implementation and Ethics
By Isabel Fischer, Claire Beswick, and Sue Newell. Journal of Information Technology Teaching Cases 2021.
Unlike Machine Behaviour, which provides a multitude of examples, this is a single AI case which illustrates how ethical considerations have to be seen in the context of commercial decisions.
In this case Rho AI, a scale-up, aims to develop an investment tool that uses machine learning and natural language processing to measure Environmental, Social and Governance (ESG) performances of organisations.
It is a reminder that even when having an ethical purpose and trying to do good, there are financial constraints and potential trade-offs that start-ups, scale-ups and also larger organisations need to consider to develop a sustainable business model in addition to trustworthy AI. The case also reminds the reader of the need for robust data as a prerequisite to robust algorithms.
5 Building AI trust: iKure + The IBM Data Science and AI Elite team tackle bias to improve healthcare outcomes
By Jennifer Clemente. IBM 2020.
This article is one of many examples where researchers claim to have used AI to reduce bias to improve healthcare outcomes.
However, this is not an endorsement of the findings. Having discussed in the previous four articles some of the concerns around trustworthy AI, it is important to remind readers that there are also ethical challenges with the non-AI status-quo.
AI solutions might have limitations, but AI proposals - especially with human oversight and ‘human-in-the-loop AI’ - might be more ethical and more effective than the status quo.
Isabel Fischer is Associate Professor (Reader) of Information Systems Management and teaches Digital Transformation on the Executive MBA and Executive MBA (London). She also lectures on Digital Marketing Technology and Management on MSc Management of Information Systems & Digital Innovation and suite of MSc Business courses.
For more articles on the Future of Work sign up to Core Insights here.