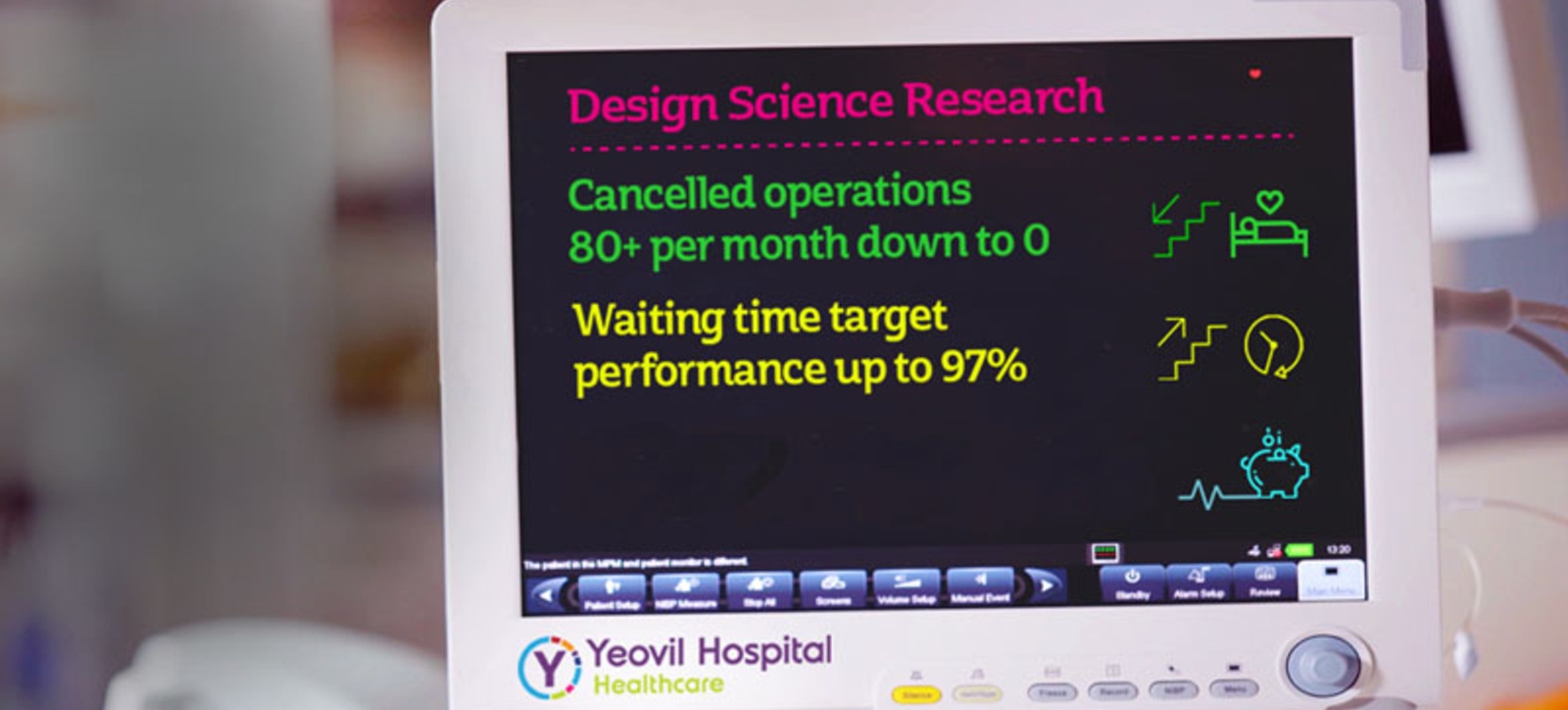
By Vinh Doan, Elvan Gökalp and Nalan Gülpinar
Every year at least 3,000 people die in the US because they can't find a matching stem cell donor, while in the UK just 69 per cent of the annual 2,000 people in need of a bone marrow transplant find a donor.
Around the world the search for donors of stem cells or bone marrow outstrips those that match on donor databases. In Turkey it is a similar story, where every year 70 out of 1,000 patients die because no donor can be found.
Stem cell donation is often the last chance for people suffering from various blood cancers like leukaemia and lymphoma. Only a third will find a match within their family, with the majority of seriously ill people relying on the kindness of strangers. Even then the donor and the recipient must have the same tissue type. As there are millions of different combinations, finding a suitable match is very complicated and timely.
Yet time is one thing blood cancer sufferers often don’t have. With limited resources stretched, finding a donor involves searching the database for a list of possible matches. A more advanced blood test is then taken to see if there is an exact match. If none are found the search then goes international with a network of 53 countries alerted. Sometimes the process takes longer than the patient has and heartbreakingly time runs out.
Only two per cent of people in the UK are registered as stem cell donors, but persuading more people to become donors is only part of the solution as the number of successful stem-cell transplants are significantly affected by how long it takes to find a match. This is a question of having sufficient capacity to be able to timely process the blood samples of potential donors.
But increasing capacity is expensive and with resources limited, it is important to decide where to locate facilities and how to allocate resources among different facility locations efficiently. Especially as spare capacity is in effect wasted money. This is where analytics and data science can help.
We have devised a prescriptive analytics model that helps health authorities to plan capacity so they can optimise their resources and significantly reduce the number of seriously ill people who die because time runs out for them and no donor is found.
With the day-to-day supply of donors hard to predict, plus how long the matching process will take uncertain, it is challenging to allocate resources efficiently. Instead of averaging-out data obtained in the past and potentially seeing resources run out on days of high demand, we have developed an optimal decision-making model in view of various scenarios for those uncertain factors, which aims to reduce the waiting time of patients and consequently leads to an increasing number of transplantations.
Given the importance of stem cell transplantation for patients, it is essential to be able to reduce the waiting time, especially in the worst-case scenario when demand is extremely high. We used a data-driven robust queuing model based on past blood sample data to safely approximate the worst-case waiting time when it is longer than usual. This conservative approach reinforces the importance of being able to find as many suitable matches as possible in time for patients in any health condition.
By combining prescriptive analytics and data science we were able to create a model that allows health authorities to change any of the input settings to find out the optimal capacity allocation by keeping waiting times for a probable match as low as possible within their budget.
For instance, if the supply of stem-cell donors increased thanks to a marketing campaign or if the number of patients needing transplantation started to rise, this can be inputted into the model under various scenarios to obtain the maximum number of successful searches for a match with the minimum waiting time for a given budget limit.
We looked at Turkey as a case study and investigated what sort of increase in capacity would enable the healthcare system to meet demand and reduce the number of people dying without a stem cell donation. Our model came up with a better way of allocating resources to the various donation facilities and suggested a 10 per cent increase in the number of successful searches compared to the common approach implemented in practice. This means that 59 more lives would be saved each year.
The model shows how analytics in conjunction with data science can help healthcare authorities to not just optimise their current capacity but to present a powerful argument to governments for more funding.
In fact, charities, health bodies and policymakers can use this model to argue their case for an increase in budget, arming them with evidence on how many lives would be saved with extra resources and funding.
Further reading:
Gökalp, E., Gulpinar, N. and Doan, X. V. (2020) "Capacity planning for networks of stem-cell donation centres under uncertainty ", Production and Operations Management, 29, 2, 281-297.
Vinh Doan is Associate Professor of Operations and a Turing Fellow at the Alan Turing Institute. He teaches Optimisation Models on MSc Business Analytics and Decision Making Under Uncertainty on the Undergraduate programme.
Nalan Gülpinar is Associate Professor of Operational Research and lectures on Supply Chain Analytics on MSc Business Analytics and Financial Optimisation on the Undergraduate programme.
Elvan Gökalp is Assistant Professor at University of Bath after completing her PhD at Warwick Business School.
For more articles on Healthcare sign up to Core Insights here.