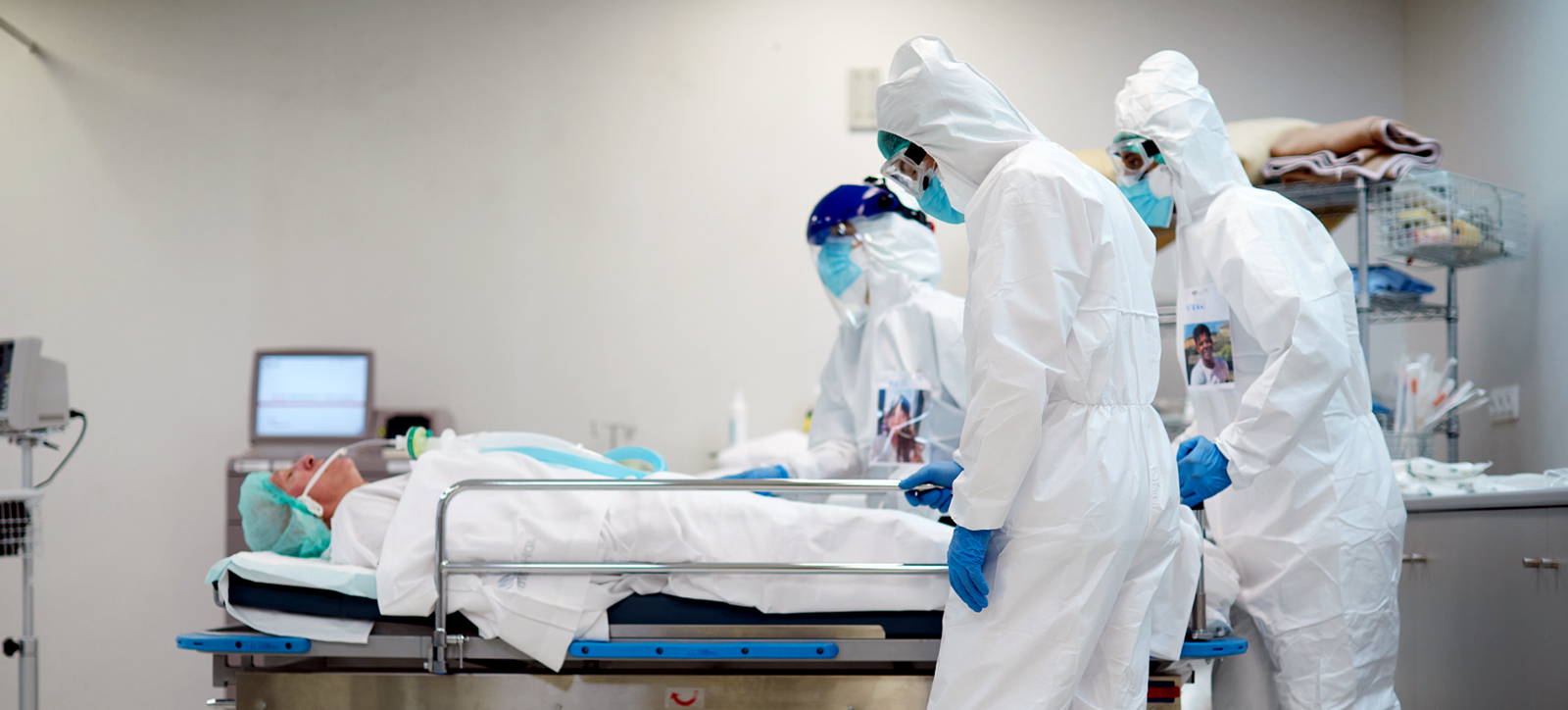
As the COVID-19 pandemic has highlighted a significant challenge for senior decision-makers, whether in government, business or elsewhere, is trying to accurately evaluate the diverse information on which they are basing a particular decision.
Part of that challenge is determining which evidence is relevant and how much weight to attach to it. This is particularly true, for example, in a fast-moving crisis, especially as the evidence is likely to be incomplete.
The task is not made easier given that the decision-making process can be distorted by cognitive biases – inherent flaws in the way people process information. A well-known example is 'the dilution effect', where combining irrelevant information with relevant information appears to dilute the impact of the relevant information.
In one experiment that demonstrated the dilution effect, two groups of participants were asked to decide whether a man had murdered his aunt. The first group was provided with the relevant diagnostic evidence - that the man was known to have argued with his aunt and had no alibi.
The second group was given the same evidence, but also some irrelevant non-diagnostic information - that the suspect was of average height and had normal vision. The second group expressed less confidence that the suspect was guilty of murder. The irrelevant information about height and vision had diluted the effect of the information about the argument and alibi.
This effect, which has been shown for many different decision-making tasks, has long been attributed to the addition of non-diagnostic information diluting the weight granted to the diagnostic information. However, research conducted together with my academic colleagues Adam Sanborn, James Tripp, and Takao Noguchi, reveals that this understanding of the dilution effect is incorrect. And, in light of our findings, that there a number of strategies regarding the weighing of evidence that can assist decision-makers (and also those seeking to influence decision-making processes).
Despite existing assumptions, it seemed plausible to us that another mechanism could be responsible for the difference in outcomes between judgements based on diagnostic evidence alone and those based on diagnostic evidence mixed with non-diagnostic evidence. That in particular, the effect could be caused by an overestimation of the importance of the diagnostic evidence.
Consequently, unlike previous research investigating this effect, we set out to learn more about the mechanism responsible using experiments that allowed an objective assessment of the evidence presented. We could then compare the different evaluations of "relevant diagnostic evidence alone" and "relevant diagnostic evidence plus irrelevant non-diagnostic evidence" with the objectively optimal evaluation.
Our findings were both surprising and contrary to the accepted wisdom. It transpired that participants in our experiments evaluated a combination of non-diagnostic and diagnostic information relatively accurately. The difference in the assessment of diagnostic evidence alone and diagnostic evidence combined with non-diagnostic evidence – the so-called dilution effect – was not caused by dilution after all, but by a tendency to overestimate the strength of diagnostic evidence alone. Indeed, it might be better described as an 'overestimation effect'.
Furthermore, our experiments revealed that this overestimation effect appears to be the result of people filling in gaps in the available information in a biased way when evaluating diagnostic evidence. Imagine, for example, that an HR manager is under pressure to hire a specialist to help with a crisis situation. The prospective candidate's CV appears outstanding. However, a page containing their university degree results is missing.
Does the dilution effect really exist?
If the HR manager wants to make a decision without obtaining the missing information, a sensible way to proceed would be to score all the degree possibilities – from first class honours to an ordinary degree – and integrate an average of those scores. That way the uncertainty inherent in the missing information is preserved when assessing the evidence.
In reality, though, people struggle to simultaneously consider a range of different, conflicting evidence. There is a tendency to fill in missing information in a biased way, based on what a person considers most likely to be true. If the rest of the CV is favourable the HR manager might well make a biased best guess and assume the candidate went to university, did well, and got at least an upper second, ignoring other possibilities.
The findings show that decision-makers are highly likely to fill in information gaps in a biased way, overestimating the importance of the diagnostic information available. As a result they may well take action with greater certainty and conviction than is warranted.
Given the number of critical decisions based on incomplete information it is prudent for decision-makers to be aware of this tendency. Remedying this bias is not simple, though. Merely knowing such a cognitive bias exists is not enough to avoid being affected by it. However, there are some steps that decision-makers can take, in the light of our findings, to improve the quality of their decision-making.
For a start, our work shows that people are poor at integrating different sources of evidence together to provide a single judgement. An expert making a decision, for example, tends to be very good at identifying information that matters, but far less good, well below optimal, at integrating all of that information together in order to make the best decision.
Generally, when judging evidence to make a decision it would be better to let experts decide what matters and allow a machine or algorithm to calculate how much it matters.
In the absence of a suitable machine or algorithm, though, there are still useful strategies a decision-maker can deploy. When filling in gaps in relevant information as part of the decision-making process it is possible to consciously avoid resorting to biased intuition. Instead, the decision-maker should force themselves to stop and evaluate what they consider both the likely and unlikely possibilities - to consider the course of action they would take for all counterfactuals for the missing information, not just the most likely. This should provide some protection against the overestimation effect.
Interestingly, the findings also suggest a strategy that can be used by organisations and individuals to influence decision-makers. If incomplete information is presented to a decision-maker they will make assumptions about the missing information that fits the picture they believe most likely. It is easy to see how organisations could turn this to their advantage by omitting information that does not suit their own agenda or objectives, whether selling consumer products or avoiding a lockdown in a pandemic.
In the fast-paced world we live in, many important decisions are necessarily based on incomplete information. But with a better understanding of how we evaluate relevant and irrelevant information, at least we may be able to limit the extent to which our judgement is skewed in these circumstances.
Further reading:
Sanborn, A. N., Noguchi, T., Tripp, J. and Stewart, N. (2020) "A dilution effect without dilution : when missing evidence, not non-diagnostic evidence, is judged inaccurately", Cognition, 196, 104110.
Sanborn, A.N. & Beierholm, U.R. (2016) "Fast and accurate learning when making discrete numerical estimates", PLoS Computational Biology, 12(4), pp. 1-28.
Neil Stewart is Professor of Behavioural Science and Business Statistics on the MSc Business Analytics and Behavioural Science and Big Data on MSc Global Central Banking & Financial Regulation.
For more articles on Behavioural Science sign up to Core Insighs here.