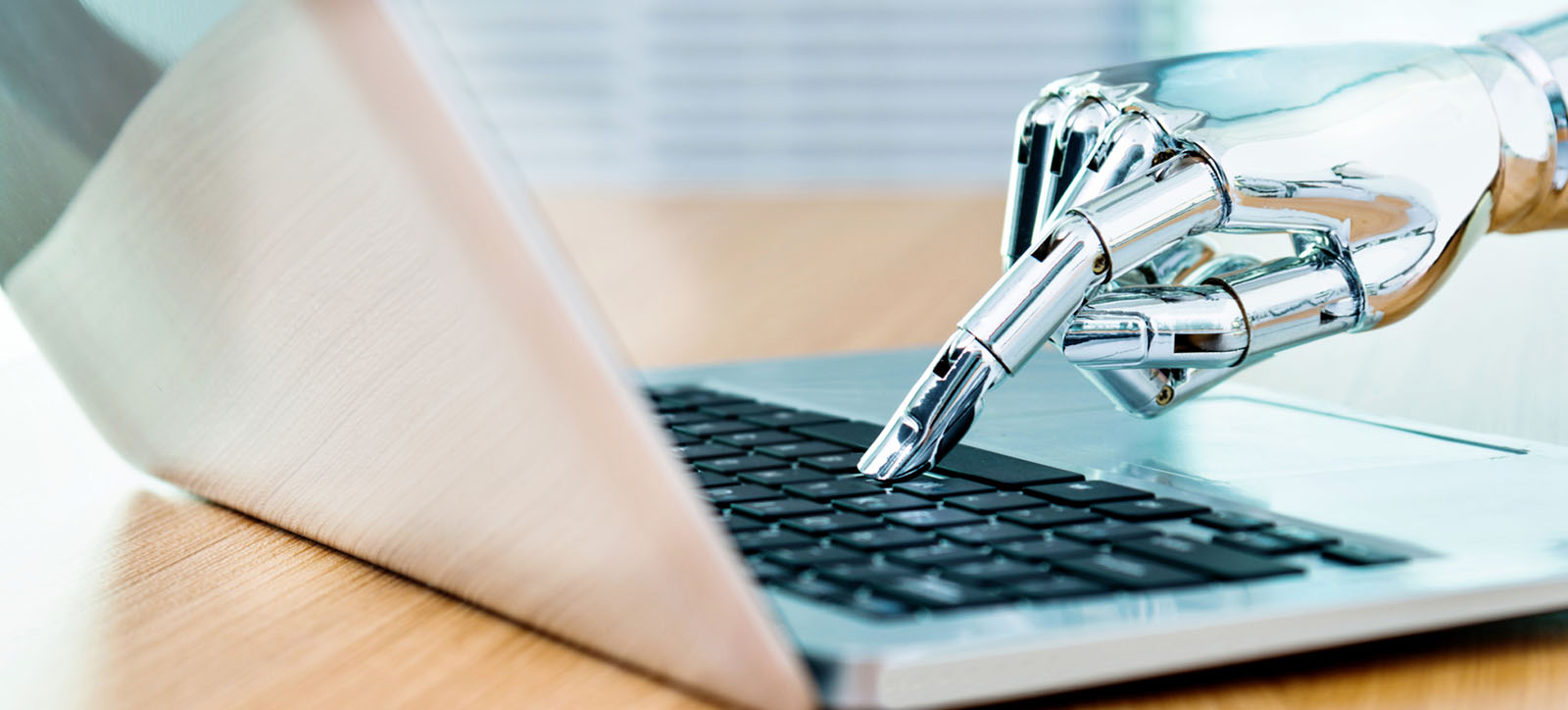
Artificial intelligence (AI) has disrupted most industries in recent years and the financial services sector has not been an exception to this development.
With the advent of Fintech and its emphasis on the use of AI, the sector has experienced a revolution in some of its core practices.
Each day billions of shares are traded on stock markets around the world and for the vast majority not a human eye, hand or brain has been involved. According to investment bank JP Morgan, passive funds and exchange-traded funds run by AI machines control roughly 60 per cent of US equity assets, while quantitative funds, which use rules-based models instead of fundamental research, now account for 20 per cent, meaning 80 per cent of stock trading is unseen by humans and in the ‘hands’ of computers.
There are three major areas of asset management in which AI plays a role: portfolio management, trading and portfolio risk management.
Portfolio management involves making decisions on the allocation of assets to build a portfolio with specific risk and return characteristics. AI techniques improve this process by facilitating fundamental analysis to process quantitative data, images or textual data and generate new investment strategies.
Essentially, AI helps produce better asset return and risk estimates and solve portfolio optimisation problems. All these result in AI achieving portfolios with better performance compared to traditional approaches.
Another popular area for AI applications is trading. Nowadays, the speed and complexity of trades have made AI techniques an essential part of trading practice. Algorithms can be trained to automatically execute trades in microseconds on the basis of trading signals, which have given rise to a whole new industry of algorithmic (or algo) trading.
In addition, AI techniques can help minimise transaction costs. Many traders have started using algorithms that automatically analyse the market and subsequently identify the best time and amount to trade.
Since the 2008 financial crisis, risk management (and compliance) have been at the forefront of asset management practices. With the increasing complexity of financial assets and global markets, traditional risk models may no longer be sufficient. Here, AI techniques that learn and evolve through the use of data can help in monitoring risk.
Specifically, AI approaches can extract information from various sources of structured or unstructured data more efficiently and produce more accurate forecasts of bankruptcy and credit risk, market volatility, macroeconomic trends, financial crises and more than traditional techniques. AI also assists risk managers in the validation and back-testing of risk models.
AI techniques have also started gaining popularity in new practices, such as robo-advising, which is where computer programs provide investment advice tailored to the needs and preferences of investors.
Will AI take over finance completely?
The popularity of robo-advisers stems from their success in democratising investment advisory services by making them less expensive and more accessible to unsophisticated individual investors. It is a particularly attractive tool for millennial and tech-savvy investors. AI can be considered the backbone of robo-advising algorithms, relying heavily on the applications of AI in asset management as discussed above, but also on natural language processing.
The use of AI has many advantages. Compared to human decision-makers, AI tools can process much larger amounts of data, and make much faster decisions. They are also not driven by personal interests, don’t become tired and are cheaper in operation.
Compared to classical statistical techniques which are great at detecting simple linear relationships, AI excels at capturing complex non-linear relationships. And while classical statistical techniques usually require clean and structured data, AI is able to process all sorts of messy data, such as satellite images to infer economic activity from the light emissions in a region, images of parking lots at supermarkets to predict their sales, or social media messages to identify trends in society.
Still, completely relying on AI seems dangerous. AI models are often opaque and complex, making them difficult, if not impossible, for managers to scrutinise.
AI models also need huge amounts of data, and are highly sensitive to the data they have been trained with. Any bias in the data may lead to bias in the AI’s decision. And while AI is great at recognising patterns in data and identifying similar situations observed in the past, it is usually at a loss on how best to act in new and previously unseen situations, such as the COVID-19 outbreak.
This is compounded by the fact that the quick and often self-reinforcing interactions between multiple AIs increases the risk of systematic crashes. And while deploying AI is usually cheap, their development and implementation costs may be considerable.
AI also faces several challenges that are unique to finance. First, while the breadth of data that can be used in finance is quite large, the time series are often very short and usually limited to a few decades. A limited number of time series observations means that any model using the data is also constrained to be proportionally small.
Even in exceptional cases where data is available in high frequency, the data has a very poor signal-to-noise ratio, especially when the data frequency is high. Furthermore, financial data changes and evolves over time, as do the financial markets.
There are, of course, economic logics that do not change over time and that underly the markets’ behaviours. However, most machine learning models - a subest of AI algorithims that improves as they ingest more data - are so-called black boxes and do not provide any insights regarding how they produce specific results. This lack of interpretability makes it difficult to understand whether a machine lerning model is capturing economically meaningful patterns or pure noise.
Despite the current hype, AI is still in its early days in finance and has a long way to go before it can replace humans in all aspects of asset management. So far, AI is mostly limited to automating specific tasks within asset management, often with some form of human intervention at the implementation stage.
What has led to the recent hype is the availability of vast amounts of new data and the computing power to extract information from them. One can imagine that AI’s footprint will only increase as asset managers compete for more information at higher speeds.
Further reading:
Bartram, S. M., Branke, J., & Motahari, M. Artificial Intelligence in Asset Management (2019). WBS Finance Group Research Paper, Available at SSRN: https://ssrn.com/abstract=3510343.
Stein, M., Branke, J. and Schmeck, H. (2008) "Efficient implementation of an active set algorithm for large-scale portfolio selection", Computers & Operations Research, Vol.35, No.12, 3945-3961.
Branke, J., Scheckenbach, B., Stein, M., Deb, K. and Schmeck, H. (2009) "Portfolio optimization with an envelope-based multi-objective evolutionary algorithm", European Journal of Operational Research, 199, 3, 684-693.
Juergen Branke is Professor of Operational Research & Systems and teaches Supply Chain Analytics on the MSc Business Analytics. He also lectures on Applied Optimisation Methods on the Undergraduate programme.
Mehrshad Motahari studied his PhD in Finance at Warwick Business School and is now a Research Associate in Finance at the University of Cambridge.
For more articles on Finance sign up to Core Insights here.