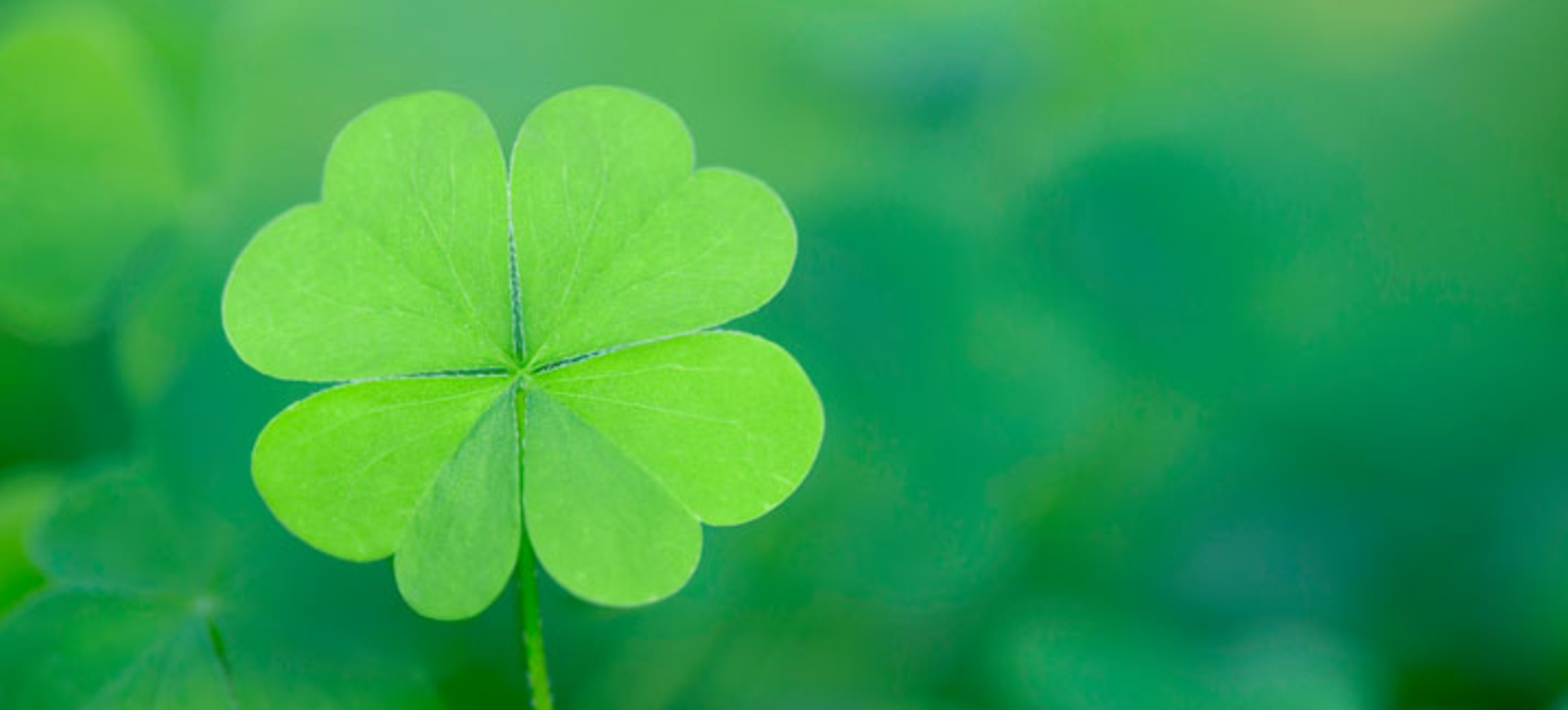
Lucky bias: Analytical behavioural strategy takes advantage of biases like luck to build a competitive advantage
No rational person would ever enter the lottery. The chance of picking the right six numbers and hitting the jackpot in the UK’s National Lottery is one in 45,057,474.
But even in something based purely on luck a strategy can be found. If it was mandatory to play the lottery how do you enhance your prospect of winning a bigger pay-out? Answer: Always pick numbers above 31.
That is because analysis has shown that the majority of people choose numbers associated with their birthday or a family member’s birthday as their ‘lucky’ numbers. So picking above 31 will ensure that if your numbers are chosen you will get a much larger slice of the winnings.
This kind of contrarian thinking can be applied to business as well, where strategy and behavioural science can be combined to exploit the many seemingly irrational biases we all have. It is something I have been researching for nearly a decade and teach on our Executive Diploma Series.
I show how recognising biases that we have, fixing your own and then exploiting others can lead to a successful strategy for business. How exactly you do it needs solid evidence and analysis to provide a strong foundation for strategising.
Hence, I call my approach 'Analytical Behavioural Strategy', ie drawing on behavioural science knowledge to search for contrarian opportunities and then utilising data analytics to formulate a specific exploitation strategy to gain a competitive advantage.
For instance, most people don’t recognise regression to the mean, which can be used to quantify the impact of luck on performances. Regression to the mean happens whenever a performance is not entirely under the control of the person or organisation, such as sales performance or firm growth.
A great performance suggests the managers in charge are better, but also indicates greater timing or luck. By definition luck is not going to persist, so their future performance will be not as great as their current performance, ie regressing downward to the mean. The good thing for a contrarian strategist is that many rivals will naively assume that the great current performance will persist.
Let’s look at the music industry. If a new band or musician has a top 20 hit should a music label immediately try to sign them? My analysis of 8,297 acts in the US Billboard 100 from 1980 to 2008 would suggest not. Music label bosses should instead be looking to sign up those reaching between 22 and 30 in the charts.
My analysis shows that for those charting in the top 20 their next single will land between 40 and 45 on average, regressing disproportionally more to the mean than their lower performing counterparts.
Those charting between 22 and 30, meanwhile, have the highest predicted future rank for their next single. This is where music label bosses will find the hidden gems.
Most of their rivals will be bidding for those superstars who entered the top 20, which are both more expensive and have lower expected future performance. In contrast, looking at the 'second best' should unearth cheaper acts that are actually going to produce more impressive future successes.
Another domain this can relate to is hiring. All companies rely on attracting top talent, none more so than universities. Typically, if an academic can publish in one of the recognised world elite journals they can demand a premium wage from universities.
But when I evaluated 1,100 leading journals across natural and social sciences, I found that high number of citations do not persist. If a journal published an exceptionally highly cited paper - higher than a cut-off around 500 citations - its next volume’s expected citation regresses disproportionally down to the mean.
The implication is that the additional citations received beyond the cut-off are 'undeserved': these extra citations should not be attributed to the journal’s superior quality, but should instead be due to the 'Matthew Effect', that is when eminent scientists will often gain more credit than a comparatively unknown researcher, even if their work is similar.
Here is the problem: these leading journals tend to acquire their elite status by having a high impact factor, but the impact factor is sensitive to exceptionally highly cited papers. My results show that these 'outliers' do not indicate superior quality.
Hiring policies solely based on counting the number of publications at these elite journals are as if universities are rewarding good luck. Universities could use my approach to pick up undervalued talents, such as those academics who publish in journals with a less exceptional impact factor in their field but do so consistently. Otherwise universities not only overpay some academics for their luck, but will inevitably be disappointed when the hired stars academic's performance regresses to the mean.
Another question growing businesses face is which markets to export to? Naturally companies head to those Asian markets with a high GDP growth rate like China or India. The problem with such a strategy is that most of their competitors will be heading for those countries as well.
A careful analysis of GDP growth around the world reveals that regression to the mean is also very strong but has an asymmetrical effect. In this case, it is at the bottom where the hidden gems could be. If a country has a very poor growth rate - that is in the bottom 10 - it will perform significantly better than the second worst countries, those in the next 10 worst performers.
The contrarian company will profit from being one of the few, if not the only, one investing in these countries. It is a brave move, but sometimes the wisdom of the crowd has to be balanced against the strong competition you will face in high growth countries or industries.
I have found that it is countries in the lowest 10 per cent - producing growth around -3 per cent - that are predicted to improve their GDP growth rate substantially. In fact, the bottom 10 are expected to regress upward to around the 45th percentile in the year that follows. Many will have terrible prospects due to wars or crises, but there might be some that are under the radar due to political reasons and still have a reasonable economic future.
A good example is China after the 1989 Tiananmen Square protests, which sparked worldwide condemnation and saw many Western companies pull out of the country. Instead of following the consensus many Taiwanese and Hong Kong companies moved into China and their investment was welcomed with open arms. They gained first mover advantage, which has helped them keep ahead of a sway of Western firms ever since.
This shows how being aware of the biases discovered in behavioural science like herding can help companies stay one step ahead of the competition and create new strategies to take advantage of rivals’ blind spots. Fortune favours the strategists who understand this theory and embrace what the evidence suggests.
Find out more about Analytical Behavioural Strategy on the Executive Diplomas Series.
Chengwei Liu is Associate Professor of Strategy and Behavioural Science.
Follow him on Twitter @ChengweiLiu.
For more articles on Strategy and Organisational Change sign up to Core Insights.