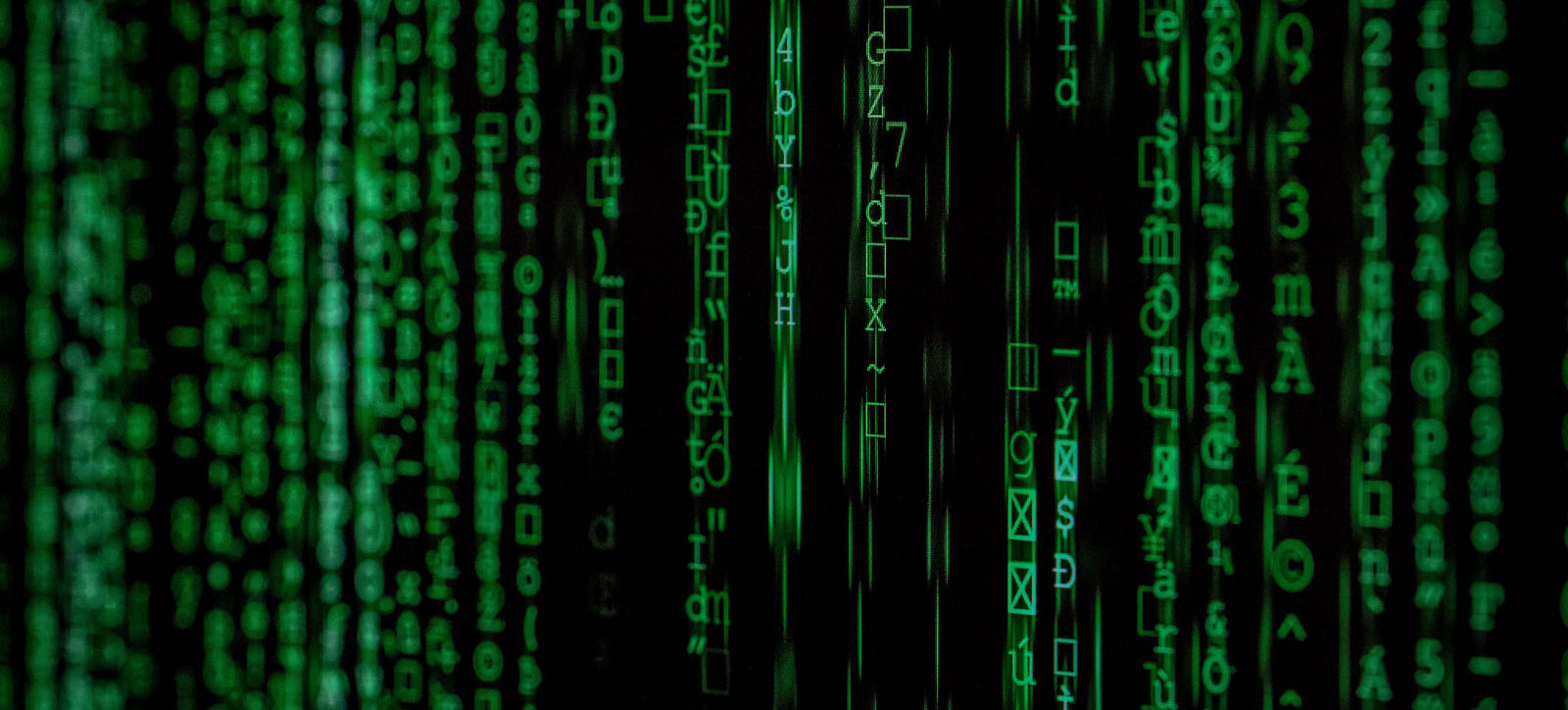
By Ola Henfridsson, Robert Gregory, Evgeny Kaganer and Harris Kyriakou
Although the phrase ‘network effects’ has become popularised in the business world thanks to the emergence of social media and the exceptional exploitation of it by the likes of Facebook and Twitter, it has been known about since the early 1900s.
That was when the telephone emerged and Bell Telephones managed to build a big enough system to reap the benefits of network effects. Nowadays it has become a central component of any aspiring technology platform’s strategy.
What is meant by the term is the more that people use a service or product, the more valuable it becomes to each user. Facebook has been the prime example, as the more people that use the social network, the more valuable it has become to each user, since more users mean more people to interact with. And with more than one billion users that is certainly a huge network effect, which has attracted the attention of billions of dollars of advertisers’ money gaining users an indirect network effect.
By using AI to mine the mountain of data platforms, such as Facebook, Uber and Airbnb are collecting a new category of network effect has emerged – data network effects. This is where the more that the platform learns from the data it collects on users, the more valuable it becomes to each user. For example, the more that Google learns about users and the searches they conduct, the more it can personalise the experience, making the search engine more valuable to each user, while the more songs people listen to on Spotify the more it is able to narrow down its recommendations and the more valuable it becomes to the listener.
The more valuable the platform becomes to the user the more likely they are to stay and keep using the service and the more personalised it will be – it’s a virtuous circle, which AI facilitates.
Thus, somebody’s enjoyment and value gained from a tech platform is directly related to the scale of the data-driven learning and the ability of its AI system. The more data and better AI, the more functionality, platform quality and better experience for each user.
But how does a company achieve these data network effects? Our research has unearthed three dimensions that are vital ingredients in creating this effect.
1 Platform legitimisation
When building a company’s AI capability the responsible use of data and ensuring that any decisions it makes are easily explainable to users is a key strategic consideration. Systems and processes to secure users’ data and privacy must be in place if the platform is to build trust and be accepted as a legitimate part of society.
Assuring users their data is secure is of prime importance when building data network effects, as any data security breaches, privacy violations or unexplainable behaviour from the AI that alarms users will damage the platform’s reputation and turn people away, thus devaluing its data network effects.
Gaining legitimacy with key stakeholders, including regulators, is vital for a platform to acquire access to the personal data, investor finance, skilled employees and technological capabilities needed to set up machine learning algorithms, train AI models and develop new personalised features.
Data privacy and security have to be part of the building of the platform from the start and central to each step. There needs to be clearly written policies, which can be easily checked by regulators, investors and any other stakeholder. If these policies and processes are not transparent then the company can face a backlash just as Facebook did when it emerged that political consultants Cambridge Analytica had downloaded large amounts of personal data from the platform without users knowing.
Some AI models using neural networks make decisions that are unexplainable, so platforms should steer clear of these ‘black box’ algorithms and use AI that can be explained to gain legitimacy with stakeholders.
In order to attract sufficient user numbers and investors the predictions made need to be understood. When making loan decisions the Bank of America shows all applicants their credit score and explains the important components that are calculated by its AI to help customers understand the decision.
2 User-centric design
Without a well-designed website that puts the user at the centre then it is unlikely sufficient numbers of people will be attracted to create data network effects. And in today’s digitally-driven world, platforms need to allow users to co-create their experience, just as they do by adding photos and posts to their Facebook page, or making playlists and re-styling their Spotify account.
This is what we term consumerization, where the line between consumption and production is blurred, with users becoming both – so they are ‘prosumers’. User-centric design plugs into this trend and sees firms constantly researching and trying to understand their users’ needs to adapt their platform accordingly.
By empowering users to co-create they will freely offer more personal data if they see value in it, which will help further tune the capabilities of the AI model driving the platform, making better predictions and offering the opportunity to develop more features.
Driving up user engagement is key for any platform as it helps the AI model make more accurate predictions. Every user interaction offers the company an opportunity to test certain features of a product or service and, therefore, to improve the prediction models created by machine learning algorithms to make the user experience more personal. Netflix, for example, regularly runs up to 50 experiments per user, all aimed at making personlising the experience further with better recommendations and developing new applications.
Thus, users need to be told, or induced, to use the platform frequently and understand that is how they will get the best out of it. Only by users realising what is expected of them will they do this, they need to know how using the platform more will improve their own experience.
And the easier the platform is to use, the more likely it is that people will use it in a committed way, allowing for further data-driven improvement of the underlying AI models and creating more value for each user.
The less effort required by the user the better. For example, Apple’s Siri may use complex deep neutral networks and hybrid emotional inference models as well as natural language processing, but users operate the system with simple voice commands that require little effort or the need to know about the convoluted technology behind it.
3 Data stewardship
The quantity and quality of data is vital if the platform’s AI model is to have the level of accuracy in its predictions and speed needed to satisfy today’s tech-savvy users. Thus, managing a firm’s data, collecting it, and making sure it is presented in a uniform fashion so the AI model does not have any problems digesting it, is incredibly important.
Good data stewardship fuels the AI engine. If the data is not managed well it can slow predictions down or produce errors. For example, IBM’s effort to train machine learning to diagnose cancer and recommend treatment options has been badly hampered by handwritten notes and the use of acronyms.
Similarly, if the dataset used to train the AI model is not large enough and does not contain a wide enough range of possible cases it will probably lead to the misinterpretation of new emerging patterns.
The quality of the data is dependent on its truthfulness, which we define as the degree of conformity between the recorded value and the actual value; completeness, which is where the values appear consistently over time; consistency, where the data is measured the same way each time; and timeliness, the data is updated quickly if there is any change.
By mastering these three dimensions firms have a greater chance of enabling their platform to provide great value to its users and so achieve data network effects.
Further reading:
Gregory, R. W., Henfridsson, O., Kaganer, E. and Kyriakou, H. (2020) "The role of artificial intelligence and data network effect for creating user value", Academy of Management Review.
Hukal, P., Henfridsson, O., Shaikh, M. and Parker, G. (2020) "Platform signaling for generating platform content", MIS Quarterly.
Constantinides, P., Henfridsson, O. and Parker, G. (2018) "Introduction—Platforms and infrastructures in the digital age ", Information Systems Research.
Ola Henfridsson is WBS Distinguished Research Environment Professor and Professor of Business Technology at Miami Herbert Business School.
Follow Ola Henfridsson on Twitter @OHenfridsson.
Robert Gregory is Associate Professor of Commerce at the University of Virgina.
Evgeny Kaganer is Visiting Professor of Information Systems at IESE Business School and Dean for Academic Affairs at the Moscow School of Management.
Harris Kyriakou is Assistant Professor of Information Systems and Analysis of Business Problems at IESE Business School.
For more articles on Strategy sign up to Core Insights here.