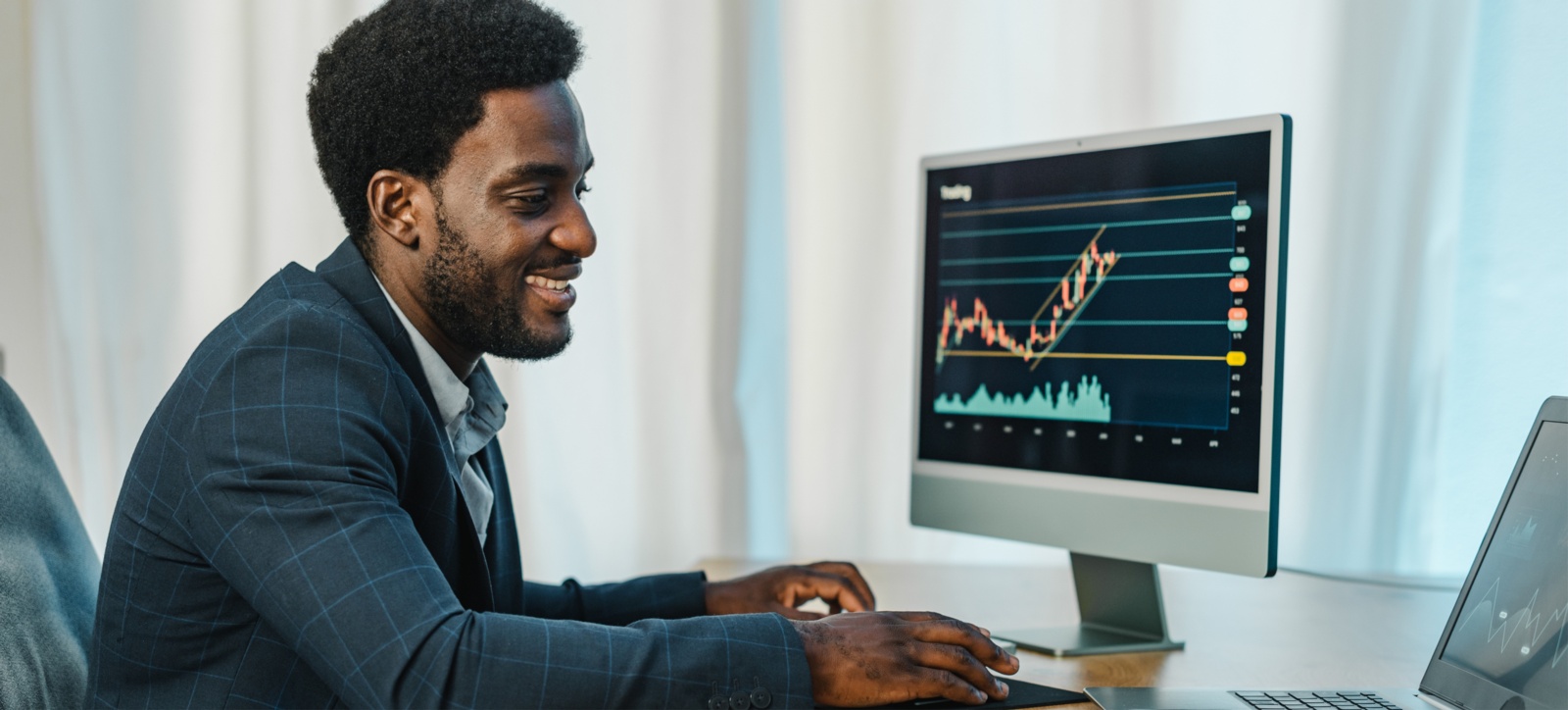
Predictive analytics is the future of sales
Anybody who has ever worked in sales or around salespeople knows that they usually guard their leads very carefully.
Indeed, they are rarely enthusiastic about sharing details about their customers with anybody, let alone entering data into a CRM database.
Typically, salespeople often consider that they are the ones that ‘own’ the customer, not the company. In fact, they’ve probably chosen sales because they are the type who like autonomy and they’d rather be out selling than uploading leads onto a database.
Perhaps this is one of the reasons why the sales sector has been slower to take up the use of data and analytics than marketing in general. Also, given that many sales transactions involve conversations of some type sales forces usually have far more complex and unstructured data to handle than marketing.
And yet despite these cultural issues it is possible to modernise sales teams with some simple analytical tools. Indeed, a new generation of sales people are making it a necessity for every company to invest in some form of analytics.
The nature of sales is changing and it’s arguably more critical than ever. Today’s customers are more informed than ever. They typically arrive at a sales call armed with exactly what type of product they need having done plenty of research online beforehand.
This means much of the value in a sales transaction comes from ongoing agreements and not one-off purchases. This can be seen in many areas that were once dominated by individual high-value transactions.
Take productivity software; once too pricey for many individuals to afford, resulting in the rise of software piracy in the 1990s and 2000s, now most of the same software is bought as-a-service subscription rather than a one-off purchase.
Many other big-ticket items are sold in this way today, with most value coming from ongoing servicing, warranties, insurance, and so on. This means the relationship between the sales person and the customer lasts longer and is more important – sales must convince a customer over time that they are still the right provider.
To help develop long term sales relationships there is more that can be done with technology than ever before. Tools that analyse sales conversations and interactions are now extremely sophisticated. Natural language processing (NLP) tools can convert free-flowing speech and online chat into a useful format.
Technology can now analyse elements of conversations such as tone, stress levels, syntax, hesitation, and emotions. Indeed, a host of companies have sprung up in the last five years with tools to assess conversations and this has only grown with the explosion in online sales chats during the COVID-19 pandemic.
With such data it is now feasible to generate the probabilities of a successful sale, or when the most effective time to use the closing techniques is, and many other useful insights. Of course, companies which sell such tools closely guard their algorithms, but research I have done with colleagues has explored whether it’s possible to predict the success of a future sales call based on the success of the previous call, and whether the environment, time of day, day of the week and other factors make a difference.
In this study we tried to develop a set of metrics that could predict the success of sales based, for instance, on the result of prior calls. From bidding for contracts to an idea of which member of staff is best placed to make the next call – this is predictive analytics for sales and could be transformative.
But just because it exists, it doesn’t mean sales teams should use it. Simple is often better. Sales analytics is capable of some impressive and highly complex analyses and predictions, but the marginal gains it brings don’t always justify the complexity and cost – there’s always a trade-off.
There’s a risk too of a negative human response. A tool may be too opaque and complex for sales staff to feel they can trust it. Or it may bamboozle some to feed too much real-time information into it during a sales interaction: if you tell a sales team member a call isn’t going well or that it’s time to move in to the 'close' will they panic?
When these tools are created what actual users want and need is not always high enough on the priority list. Most sales people aren’t trained in data analytics, and if they’re not going to use a tool, it’s a wasted resource.
One of the greatest challenges with any technology is to get users to trust it and thus adopt it. Managers must focus on the usefulness of any predictive model.
There’s also a host of more simple metrics to use without the need of a complicated artificial intelligence algorithm. A simple regression model would help sales people understand what the data is telling them.
But the days when sales staff could rely on ‘gut feel’ are over - every sales force uses some form of analytics today.
When we first started researching sales analytics some 15 years ago we met experienced staff who would say: “I’ve been selling for 30 years and I know who my customers are and what they want.”
Today there is a new breed of sales reps who are more comfortable with data and technology and managers’ horizons are wider than they used to be.
Information is out there already. This will continue to change as the younger generation becomes more senior within organisations. Predictive analytics will be the future.
Further reading:
Nahm IY, Ahearne MJ, Lee N, Tirunillai S. Managing Positive and Negative Trends in Sales Call Outcomes: The Role of Momentum. Journal of Marketing Research. June 2022.
Baehre, S., O’Dwyer, M., O’Malley, L. et al. The use of Net Promoter Score (NPS) to predict sales growth: insights from an empirical investigation. Journal of the Acadamy Marketing Science. January 2022.
Nick Lee is Professor of Marketing and teaches on the Warwick Executive Diploma in Marketing Leadership. He also lectures on Marketing for the Distance Learning MBA and Executive MBA plus Customer Experience on the Undergraduate programme.
For more articles on Decision Marking and Analytics sign up to Core Insights here.