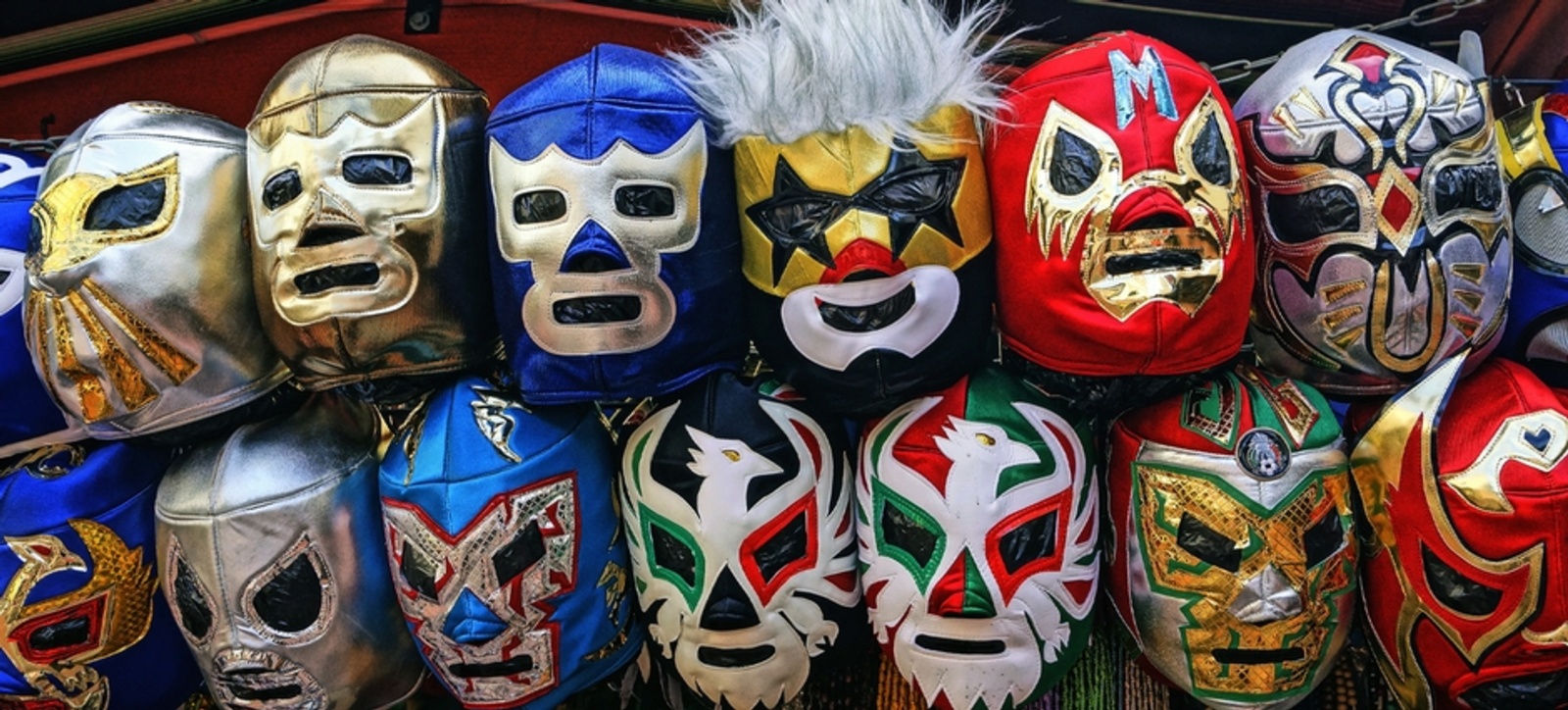
Core Insights: Future of Work
When microchip giant Intel set about creating their Fab 42 facility in Arizona, US, to manufacture tiny 10nm chips used mainly in smartphones, it was estimated to cost $7 billion.
This enormous sum not only reflects the growing complexity of modern microprocessors - 10nm means they measure around 10 nanometres, that's 0.00001 millimetres - but also the pressure placed on the industry by Moore’s Law, where computing power doubles every two years.
Given the intense complexity of designing the latest generation of microscopic microchips, it’s no surprise that designers and engineers are turning to technology to help them in their work. The dominant design approach is a traditional manual method that involves a schematic design of a portion of the chip, with computers aiding engineers as they transform the design into a physical form.
In recent years a suite of autonomous AI tools have been created that provides a powerful way of improving the productivity of this process, due in large part to their ability to generate full layout solutions for whole sections of a chip autonomously.
With chips getting ever more complex, this new approach is coming to dominate and firms are moving away from the use of more traditional tools. As firms operate with both traditional and autonomous tools alongside one another, however, this presents various challenges.
Traditionally designers have had a degree of confidence that what is produced will be based upon the inputs provided and the logic that underpins the tool. More importantly, they will know why the tool has designed the chip to be a certain way. As AI-driven tools become more widespread, however, it’s increasingly likely that designs will be made via 'black box' decisions, where engineers are far less confident in the method used by the AI tool and can't work out the reasoning.
Along with my research colleagues, we attempted to understand how this is changing the relationship between engineers and technology at a leading global semiconductor manufacturer. The company has manufactured integrated computer chips for more than 40 years and has been using technology to assist them for at least 30.
As a result, the company’s design processes are supported by a wide range of sophisticated design technologies that represent, implement, track, validate and record chip designers’ decisions. Overall, the company has digitalised its design tasks in ways that put designers firmly in control of the design process and its outcomes.
The company recently began to introduce autonomous technologies to generate an ever-greater proportion of the physical layout of an integrated circuit chip. To begin with, they only used this autonomous technology for a few parts of the chip with traditional design tech used for the remainder. The parallel use of both allowed for comparisons to be made in how engineers interacted with both approaches.
As former MIT Sloan School of Management Professor Michael Hammer famously said in 1990, a common error when thinking of technology is how it can be used to make existing processes faster or more effective. This often has limited use and greater returns are seen when processes are re-engineered to fully capitalise on the capabilities the technology affords.
This was the case with our chip manufacturer, with designers migrating from undertaking the end-to-end design themselves towards facilitating experiments conducted by the AI-based tools. In this new scenario, the designers would set up a range of experiments, let the autonomous tool conduct them, and learn any lessons from the resulting outputs before starting the whole process over again. The designers would also play a central role in the creation of autonomous tools themselves, which underlined their central, albeit modified, role.
This trend is likely to continue, as while many of the desgin autonomous tools on the market today require designers to set criteria and determine the boundaries for the experimentation that follows - with these rules typically governed by the experience and therefore limitations of the designer themselves - reinforcement learning in AI renders the machine better able to learn from its own experiments and thus operate in a more unsupervised manner.
For instance, in 2020 Google released an algorithm based upon reinforcement learning to optimise the location of the various components on a microchip that made them not only more efficient but less power-hungry.
With single floorplans of chips often taking up to 30 hours to produce, even with the assistance of design automation software, the new AI approach promises to accelerate the process considerably. This is an imperative in an industry in which speed is everything and the need for new chip architectures is surpassing the two-year life cycle the industry has traditionally operated by for each chip.
By using reinforcement learning, the system was "rewarded" whenever performances were improved, with the algorithm running through hundreds of thousands of design iterations, with each one taking a mere fraction of a second to evaluate it for the reward function. The design of the reward function was undertaken by engineers who would ordinarily be having a more hands-on role in the design of each chip. Similarly, the suggested design was also assessed and verified by expert designers.
Given the rapid pace of change in the sector, it’s inevitable that the role of technology will continue to grow in order to ensure that chips become ever faster, more efficient, and increasingly energy-efficient. Our research has already shown how roles are changing as technology plays an increasing part in the development of the latest generation of microprocessors. It’s an evolution that is only likely to accelerate as the demands of the market increase and the ability of technology to meet those needs grow in unison.
Zhewei Zhang is Assistant Professor of Information Systems & Management and teaches Programming for Data Analytics on the MSc Management of Information Systems & Digital Innovation.
For more articles on the Future of Work sign up to Core Insights here.